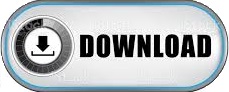
MRAE), where we use a reference forecast to scale the errors, also becomes unstable, especially when using Naïve Forecast as a reference. MAPE) become unstable because of the division by zero, which now is an almost certainty. And this wreaks havoc with a lot of the measures we have seen so far. The single defining characteristic of Intermittent and Lumpy series are the number of times there are zero demand. Unsuitability of Traditional Error Measures Typical examples are Spare Parts sales, Long tail of Retail Sales, etc. But in the real world, there are a lot more Intermittent and Lumpy timeseries. The Forecast measures we have discussed was all, predominantly, designed to handle Smooth and Erratic timeseries. Using these cutoffs, they defined highs and lows and then putting both together a grid which classifies timeseries into Smooth, Erratic, Intermittent, and Lumpy. They have defined intermittency cutoff as 1.32 and cutoff as 0.49. If is high, that means that the variability in the series is also high.īased on these two demand characteristics, Syntetos and Boylan has theoretically derived cutoff values which defines a marked change in the type of behaviour. This shows the variability of a time series. We calculate the standard deviation, but then scale it with the mean of the series to guard against scale dependency. if the ADI for a time series is 1.9, it means that on an average we see a non-zero demand every 1.9 time periods.ĪDI is a measure of intermittency the higher it is, the ore intermittent the series is.Ĭoefficient of Variation is the standardized standard deviation. They used wo parameters of the time series for this classification – Average Demand Interval and Square of Coefficient of Variation.Īverage Demand Interval is the average interval in time periods between two non-zero demand. Syntetos and Boylan(2005) proposed a more formal way of categorizing time series. SourceĬasually, we call intermittent series as series with a lot of periods with no demand, i.e. But there is a whole different breed of time series in real life – intermittent and lumpy demand. But all of them were really focused on smooth and steady time series. In the previous few blog posts, we’ve seen all the popular forecast measures used in practice.
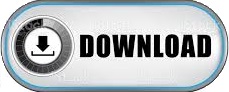